How Generative AI Can Shape The New Age Of Healthcare
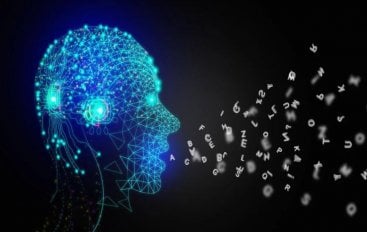
We have all witnessed Generative AI in general and ChatGPT, in particular, catching on like wildfire in the last couple of years. In fact, ChatGPT hit 10 million within 40 days of launch and crossed 100 million daily active users within two months – these are unprecedented numbers. There is significant interest to see how Generative AI will impact our lives and businesses across industries are trying to stay ahead of the curve by creating new applications that are built on ChatGPT or Bard.
Breaking it down in the parlance of technology adoption curves, Large Language Models (LLMs) have now hit the peak of inflated expectations in no time, and this is typically the stage at which hype overshadows proof and most ecosystem players are looking for practical use cases to build on the momentum. I see there is tremendous potential with Generative AI which will lead to a definitive shift in the way most industries function.
Potential impact of ChatGPT in healthcare
Usually, the healthcare industry has been a bit slow in embracing new technologies, however with Generative AI and Chat GPT, I see a lot of momentum with the use cases being created. Such enthusiasm and interest are unprecedented in the healthcare space, and I am quite excited to see how things take shape.
When traditional AI gathered pace, it was the fastest adoption of a new technology that most of us had witnessed. However, the adoption of generative AI is unprecedented, and we have hardly experienced anything like this yet.
The future is truly here and there will be a paradigm shift on at least two fronts – the way we do things and the time we take in doing them. It would not be a stretch to say that the “New programming language is the language that one speaks!” – it can be English, Hindi, Hebrew, or Arabic. And the conversation to code is going to be less than 10 seconds.
Now let’s look at some of the most pertinent use cases in healthcare that Generative AI can potentially enable –
Summarising medical records: It could be as simple as a conversation between a doctor and a patient. There are already LLM tools that can summarize the entire discussion under different buckets like allergies, prescriptions, pre-existing diseases etc. and load data into EMRs. In fact, summarizing a complex conversation of 30 minutes can be done by the time the doctor ends the call with pleasantries, so all the doctors must do is review and approve the final version.
Answering questions: LLMs can understand vast sets of materials that are provided to them and start answering questions. The answers are not just retrieval of data from stored objects, but rather it would complete reasoning-based answers with surprisingly great accuracy. You must understand that LLMs are not fact databases, rather they are reasoning databases.
Understanding biomolecular data: LLMs can be used to interpret biological and chemical data such as DNA and proteins and connect them to health conditions. This could help us enormously in drug research and development.
LLMs can be trained on omics datasets to predict novel targets and their binding to appropriate candidates, screening against large chemical libraries, fast-paced in-silico simulations to predict ADME properties and efficiently handling unstructured scientific data among many other benefits.
Diagnosis: LLMs can analyze vast amounts of medical, social, and patient-generated health data and assist in accurate diagnosis of diseases. Evidence based decision making is key to accurate diagnosis. For eg: Any subtle abnormalities in ECG could go unnoticed otherwise and LLMs can be more accurate in finding such subtle abnormalities.
Personalized treatment planning: A person could be lactose intolerant and the same might not be visible to care givers during treatment planning. LLMs can be used to build algorithms that pick up & examine a variety of patient related data elements to either forecast probability of complications due to certain treatment pathways or customize treatment protocols based on risk.
Early disease detection and risk assessment: With the amount of personal data that can be generated today – be it social determinants (SDOH) of a patient or data from wearables and other clinical ontological data, we can possibly predict certain diseases including Parkinson’s, strokes and other serious diseases or even provide predictions and alerts.
While many of these use cases can seem to be quite complex, they can be done quite efficiently using LLMs. Industry-leading hospitals and EMR companies are already working closely with LLM technology development companies to build use cases that are quite ahead in the development life cycle.
Often, the success of many businesses is dependent upon identifying an inflection point and building the capabilities required to capitalize on the resulting opportunities. We are clearly at an inflection point with the advent of Generative AI and healthcare companies would be wise to leverage its potential to provide personalized and future-ready care experiences.
We are clearly at an inflection point with the advent of Generative AI and healthcare companies would be wise to leverage its potential to provide personalized and future-ready care experiences.